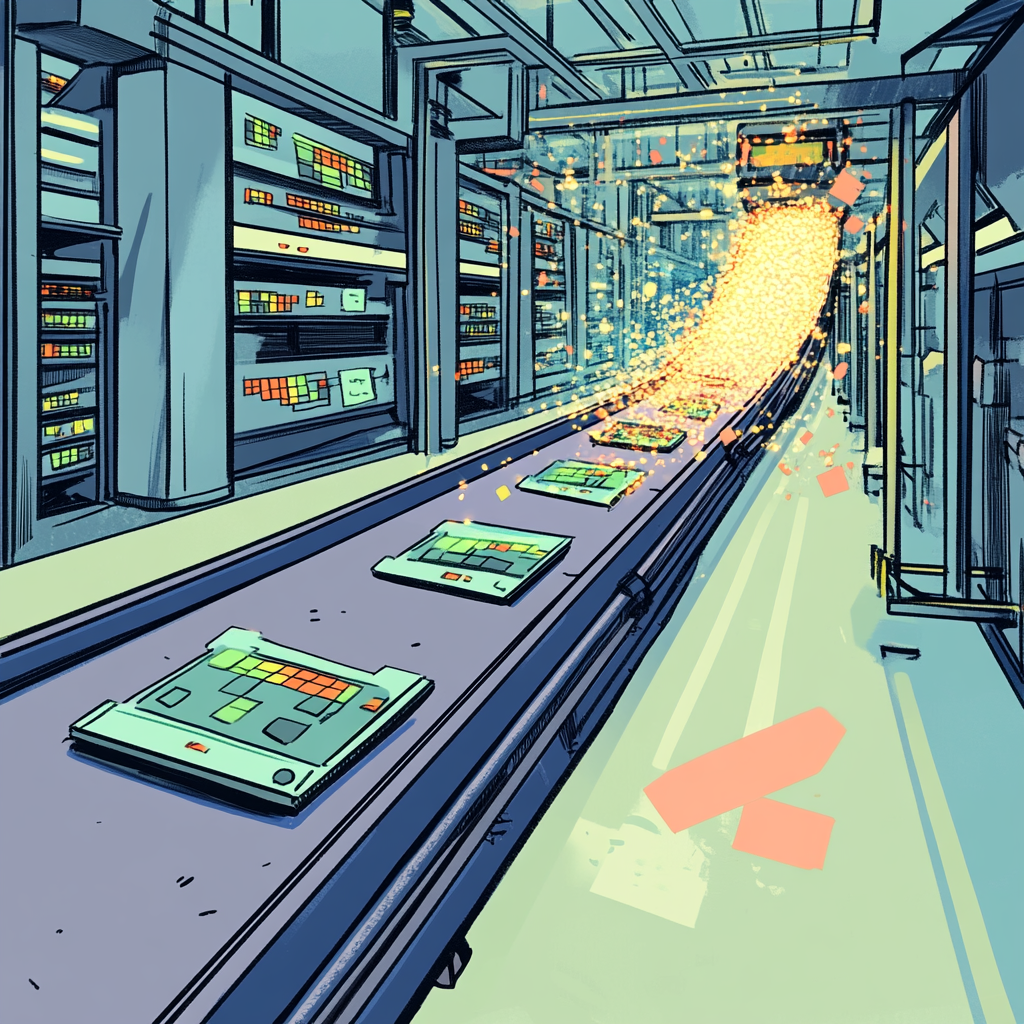
Retrieval augmented generation to enhance the accuracy and reliability of using generative AI with your data.
RAG, or Retrieval-Augmented Generation, combines information retrieval with generative models. It enhances responses by fetching relevant data from external sources, allowing for more accurate and contextually rich answers.
RAG enhances AI responses by integrating external information with the LLM. Organizations that extend models with their data can use generative AI to deep-dive context-specific information for more accurate and relevant responses. This approach improves performance in tasks like question-answering, conversational agents and providing business value.
Our RAG solutions can scale to billion+ records and we can help you achieve high-quality results which work for your data. Our team is experienced in tuning retrieval-augmented generation to deal with domain-specific queries and problems. In addition, we can leave behind simple solutions for your teams to use.
Implementation complexity abstracted.
We train your developers on how to use the tools to integrate them into your search and interactive systems.
Low developer learning curve with users left with intuitive interfaces.
Scale as much as you need with the ability to handle over a billion records.
Generative AI is changing the way customers search and interact online. Most businesses using AI assistants to respond to users need RAG to supplement an AI model’s parameterized knowledge with their data. For example –
A shop assistant who understands the customer’s tastes, trends and shopping habits.
If you have lots of information that needs to be surfaced to customers, RAG can help make it easier for you to find the right content.
A financial analyst with an AI model supplemented with market data.
A nurse or doctor with an AI assistant that has access to a medical data index.
Make your catalogue searchable more deeply and interactively, where keywords are replaced with conversations that can help with the discovery of important content.
RAG can be used to obtain database queries directly from natural language questions, opening siloed data to your less technical domain experts.
If you need to leverage the potential of combining your data with generative AI to solve difficult problems, our AI consultancy services can speed up the project. Enquire today to see about working with us.
We are AI consultants who combine our vast data knowledge with VectorLink to incorporate your structured and unstructured data with LLMs to solve challenging problems quickly.